AiThority Interview with Marc Bolitho, CEO of Recogni
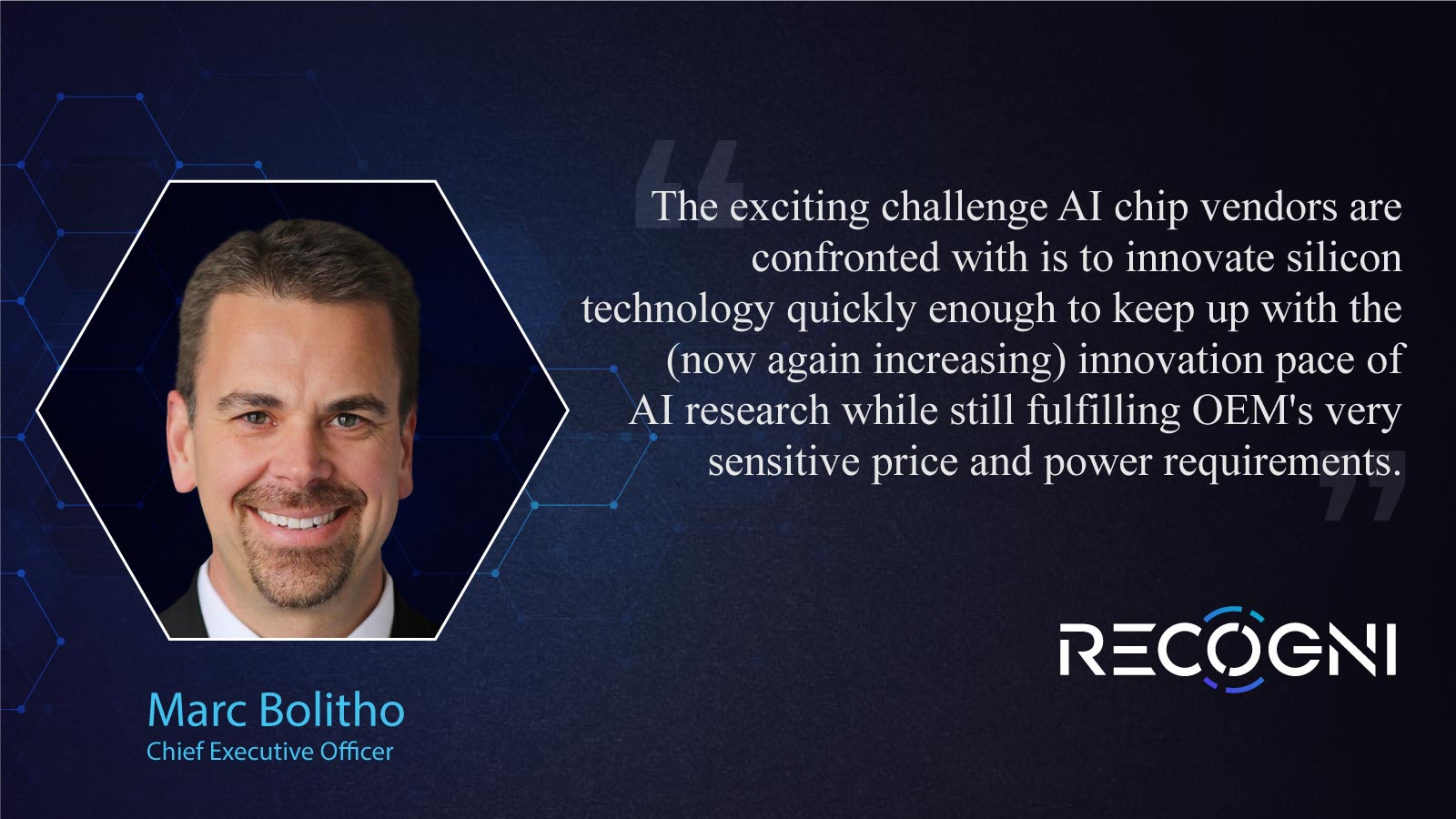
What was the biggest trend or theme that you came away with from CES?
The industry is really starting to wake up about the power consumption of autonomous systems across the different mobility spaces. Mostly the battery range discussion, but also discussions around the elimination of liquid cooling. In addition, there are concerns about the CO2 footprint of autonomous systems on vehicles and what that’s going to be in the future.
The primary concern is around range, because if you look at BEVs or EVs in general, the dominant consumption of the range is obviously the motor, the weight of the vehicle and the weight of the passengers or the freight in the vehicle. Even wiring harnesses can weigh up to 150 pounds. But, if the compute power of autonomous systems consumes a significant chunk of that, then it has implications on what’s called advertised range, what the vehicle manufacturer can advertise as range, because then otherwise, they have to fine print and caveat everything (i.e., this range is achievable only if you don’t turn on the air conditioner, don’t turn on the stereo or radio, don’t turn on the other systems that require compute power, etc.).
The point is, the value proposition of the manufacturer is that this vehicle has certain autonomous features and if it has certain autonomous features that make it safer or more comfortable for drivers and passengers, then those features need to be enabled all the time. You can selectively turn off the air conditioner, but you can’t turn off autonomous features because they enable and enhance safety and other critical capabilities. So, now the question is, if those features or those autonomous capabilities consume a certain percentage of energy, then there’s a direct impact on the range of the vehicle as a function of how long the vehicle’s driven.
What are the biggest challenges OEMs face when it comes to self-driving?
The three major trends driving the automotive industry are electrification, autonomous driving and software-defined vehicles. That challenge is that two of these (autonomous driving and software-defined vehicles) actually hinder electrification unless we can figure out the power compute problem. As OEMs look to add L2+ capabilities and ADAS features that can be added on for a subscription fee, all of these things pull from the power of the car, and in an electric vehicle, it can reduce a car’s range by 25%!
As more compute power is required to achieve levels of autonomy as well as with software-defined vehicles, we need faster, more powerful and more efficient computing to make it happen. To enable this, we will see more OEMs and Tier 1s moving to centralized computing architectures in their autonomous driving technology stacks. Centralized computing architectures allow for more efficient compute processing, especially as sensors gather higher and higher resolution data, which will continue to be a major factor in enabling safe driving and the realization of autonomous driving.
We can expect to see more Tier 1s investing in central computing architectures as well.
What will it take for autonomy to take hold?
For electric and autonomous vehicles to continue improving performance, building trust and ultimately seeing mass adoption, they need solutions that are purpose built for these vehicles. The shift to EVs makes the vehicle drivetrain architecture more simple (ie., requiring fewer ECUs) but adds new functionality needs such as exceptional battery management – which needs more powerful chips and software. We will start to see chips that are designed specifically for EVs and AVs in order to provide more power efficiency and functionality that is required by these vehicles.
The chip developers that are developing chips in China for central compute are going to have some challenges because they won’t have access to the latest technology nodes for competitiveness now. For example, a Chinese manufacturer of a central compute device may not be able to go to seven nanometers now with the latest regulations. This affects their ability to build really high performance devices needed for ADAS/AV functionality, and ultimately to be competitive from both a technology and cost point of view. It also means there’s an opportunity for new US manufacturers to be competitive or enter the game.
This also applies to GPUs and other solutions within EVs and AVs. Right now, most GPUs being used are power hungry, pulling more power from the vehicles overall system, reducing range and overall performance.
It seems everyone is trying to get into the automotive supply chain – what can we expect to see in terms of new partnerships, collaborations or new entrants?
In 2023, OEMs across the board will come to the realization that they can’t do it all themselves even. “It” being “design and build” a full autonomous driving system. They need partners. Some OEMs had the ambition to try to solve it themselves, but due to the complexity of it, there’s going to be a need for partnerships and suppliers.
OEMs & Tier1’s have limitations in attracting technical talent for great chip architects and designers. The pool of chip architects is a very limited and even shrinking group of engineers. Without this core capability, it is extremely difficult to be able to architect purpose-built solutions that differentiate and address the needs for safe autonomous systems that meet sustainability goals. Partnering with innovative start-ups and companies who are focused on developing autonomous driving solutions is the best way for OEMs and Tier 1s to achieve the goal of delivering the volume of EVs and the functionality of ADAS and autonomous features that will set them apart.
AI ML News: Making AI More Accessible: AWS & Hugging Face Partner Up To…
The tech world is experiencing record layoffs and preparing for a recession, are you seeing the same in the automotive industry?
While the tech industry is laying people off and preparing for a recession, the automotive industry is booming. And, while OEMs may have to adjust their projections for the volume of EV models they’ll be able to introduce because of material shortages, development for ADAS and autonomous vehicles continues full speed ahead and we don’t see it slowing down. It’s a race to see who can do it best and do it first. The good thing is that when we talk to OEMs, they all seem to have a much more realistic view of the evolution of autonomous vehicles – that Level 4 full autonomy is quite a few years ahead of us, but that won’t stop them from investing in development, which is great for the industry and for end user safety.
What are the biggest pain points you hear from your customers, in your sector, when it comes to AI?
As mentioned above, economical feasibility is still a big one we hear within the autonomous driving market. We are still continuing to see customers struggling with getting their ambitious plans and AI stacks into a cost-, power- and size-efficient form factor that would pave the path to wider adoption beyond prototypes that cost multiple millions of dollars. AI compute solutions alone quickly cost multiple thousands of dollars – with customers waiting for at least a 10x cost reduction until those compute solutions could reach further market penetration. One of the emerging trends because of this dynamic are centralized full-stack SoCs. Which then again come with their own challenges.
Overall, the exciting challenge AI chip vendors are confronted with is to innovate silicon technology quickly enough to keep up with the (now again increasing) innovation pace of AI research while still fulfilling OEM’s very sensitive price and power requirements. Or, to put it in other words: Lots and lots of flexible compute for reasonable price and power. Traditional chip companies thereby have a hard time following the pace as we were able to observe for example when a company announced a change in their roadmap and future product specification to catch up to ever increasing compute demand. Anti-fragility at the cost of speed is an incredibly hard landscape of compromises to navigate for any bigger company. This is where we as a startup that is living true silicon-ML-co-design on a daily basis have a big advantage.
Top AIThority Interview: AiThority Interview with João Graça, Co-founder and CTO at Unbabel
How have you seen AI/ML tech evolve over the past year; What do you think the biggest trends have been?
In our field of Perception (camera-, RADAR, and LiDAR-based vision) transformers have been the most prominent topic in 2022.
Why?
In a nutshell: They are unlocking the ability to learn and map mathematical concepts which come in very handy for solving existing problems in new and easier ways. Examples are fusion of multiple viewpoints of multiple sensor modalities without the need for a human engineer to design for instance projection concepts. Or as mentioned above, any truly three-dimensional perception of objects and structures becomes a much more elastic and implicitly learned challenge with the help of transformers. The interesting thing here is: One does not have to add a lot of transformer-compute to an otherwise CNN-based model to leverage this additional degree of freedom.
What are the biggest AI opportunities that you see coming down the pike?
As AI based implementations improve to address end-to-end functionality rather than partial solutions, we see more reliance on AI-based applications. The impetus behind such applications is a reduction of wasted time, particularly in repetitive tasks, less reliance on personnel and labor force, and more around the clock service with improved operational costs. Opportunities are in a wide gamut of applications such as full autonomous driving, autonomous mobility in service industries including last mile deliveries, autonomous planes and drones, warehousing, hospitality, industrial automation, autonomous farming, and mining/heavy industrial machinery.
What are your biggest predictions in terms of AI in 2023?
We see continued demand for inclusion of AI-centric autonomy solutions in 2023 and subsequent years. AI elements are across the entire autonomous driving software stack, inference processing, sensor fusion, and path planning. There is an increased need for more data throughput and associated AI processing which drives the compute capacity requirements. In line with upgradability, AI algorithms change over time to achieve a high level of confidence in accuracy. There is also other functionality such as natural language processing (NLP) and use of transformer models that are under consideration for the new driving autonomy platforms.
Thank you, Marc! That was fun and we hope to see you back on AiThority.com soon.
Marc Bolitho is the CEO of Recogni, the leader in AI-based perception purpose-built for autonomous vehicles. He has 28 years of experience in automotive electronics. As the Senior Vice President of ZF Group, he was responsible for the ADAS business unit with $2B in annual revenue and a global team of 5000.
Recogni provides exceptional vision-based perception processing to Autonomous Driving platforms addressing high compute, low latency, and low power consumption. The company was founded in 2017 with offices in San Jose California and Munich Germany. Lead investors are GreatPoint Ventures, Celesta Capital, Mayfield, DNS Capital, as well as notable automotive OEM and tier 1s including BMW iVentures, Toyota Ventures, Bosch, Continental, Forvia and FluxUnit-OSRAM Ventures.
Comments are closed.