AiThority Interview with Joseph Mossel, CEO at Ibex Medical Analytics
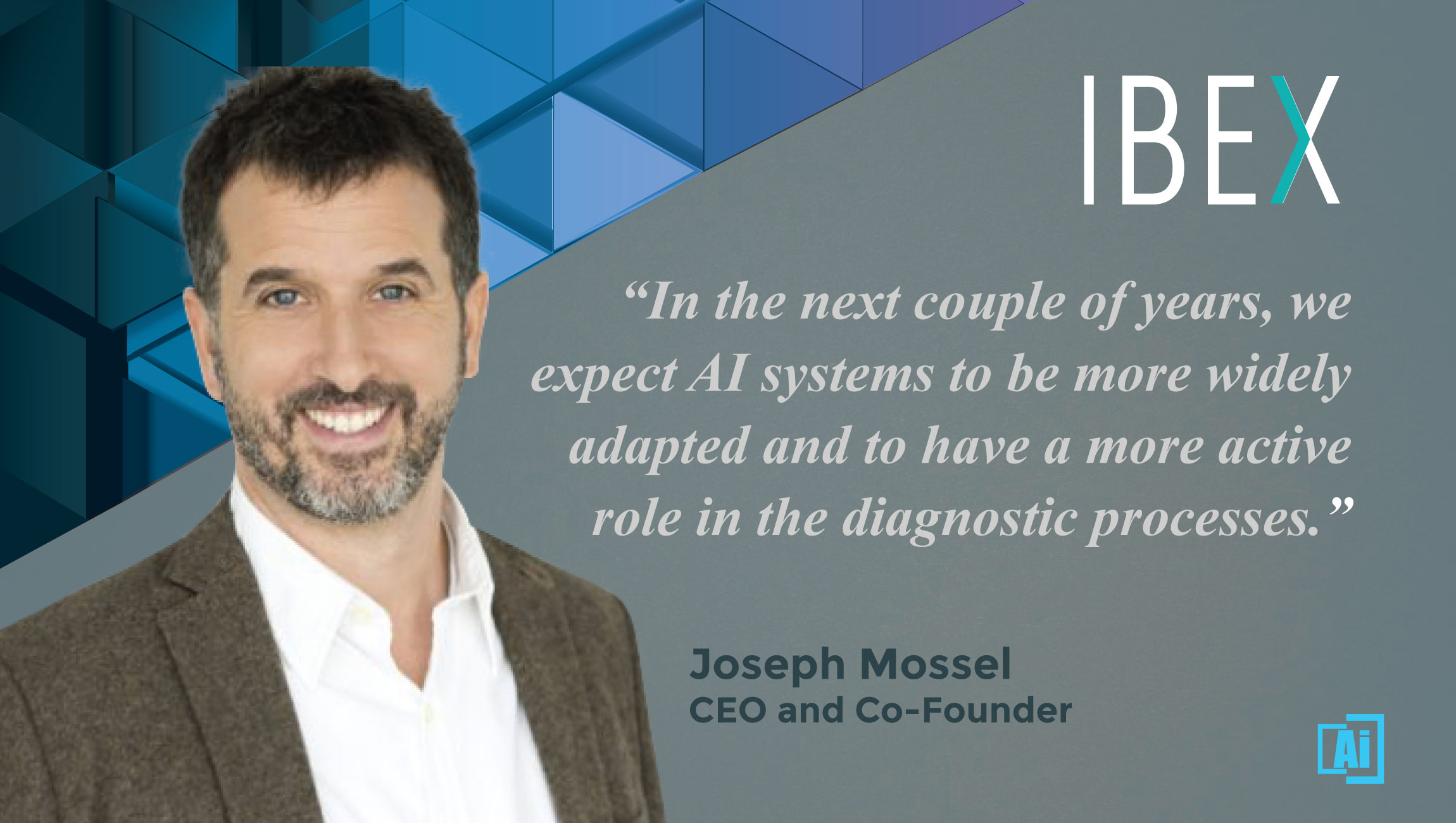
Tell us about your journey into AI and Medtech, and how you started Ibex Medical Analytics.
My co-founder, Dr. Chaim Linhart, and I both have extensive experience in Software Engineering and Machine Learning and were looking for new ways to apply Artificial Intelligence (AI) in Healthcare.
During a family dinner with a relative, who is also a Pathologist, I learned that although the pathological analysis was still carried out predominantly on physical tissue samples through microscopes, a new trend toward digitization was starting to pick up among pathology labs. We realized that if physical samples were now being replaced with digital images analyzed by a human pathologist through a computer screen, then there is an opportunity to use AI for developing algorithms that can automatically detect cancer and other medical conditions.
The algorithms were developed by feeding Deep Learning networks with images of annotated prostate biopsy slides. After a meticulous and iterative training process involving data scientists and pathologists, our newly founded company had a clinical-grade algorithm that could detect and grade prostate cancer as accurately as a human pathologist.
Why did you choose to focus on pathology and cancer diagnosis/misdiagnosis?
First, we need to explain why pathology is so important. Pathologists have a very unique and specific skill set, detecting and diagnosing various types of cancer. However, in spite of this, pathology has not gone through much innovation over the years. For example, pathologists are still using mainly microscopes.
Additionally, there is an ongoing shortage of pathologists worldwide, but an increase in cancer rates. This growing gap, paired with new AI and Machine Learning techniques and technologies, provided us with an opportunity to help not only pathologists, but cancer patients, and the healthcare system, by helping to improve diagnostic accuracy and lower costs while streamlining the process.
How has AI and telemedicine evolved in the past five years?
I don’t think there was much going on in the telemedicine field five years ago. However, now, there are many vendors and healthcare providers that offer telemedicine.
In terms of AI, the Deep Learning revolution began roughly a decade ago. Specifically, in image analysis, the results on ImageNet (a large repository of labeled images, used as a benchmark for algorithms) were still not up to par 9 years ago; indeed, analyzing images, as well as text (language), served as good examples of how limited the capabilities of computer algorithms are relative to humans.
All that changed within a couple of years. In 2015, algorithms surpassed average human accuracy on ImageNet. The same year, the best computer programs were no better at playing GO than an amateur human; two years later, AI reached superhuman ranking. In Computational Pathology, the first algorithms that gave reasonably accurate diagnostic information for WSIs of H&E slides appeared about 5 years ago. The first successes were on relatively simple tasks and small, limited datasets.
More importantly, these were “narrow AI” (AKA “weak AI”) – models that were trained to perform a single, specific task. In the past five years, AI has transitioned in many fields from very narrow models to broader, more general ones. As an example, Ibex has recently demonstrated an AI system capable of identifying almost all cell types and structures in a prostate CNB (cancer of different types and grades, pre-cancerous glands, other clinical findings, and even various normal tissue structures), unlike earlier models that were trained only to detect adenocarcinoma (the most common cancer type in the prostate).
Furthermore, the accuracy of this system was validated in clinical studies on huge external datasets in multiple pathology labs – the system achieved clinical-grade accuracy for several diagnostic tasks. This is an example of the first steps AI is taking towards more accurate and more general, human-like Artificial Intelligence
Read More: AiThority Interview with Frederic Beneteau, VP – Head of Customer Business Team at Nokia
What challenges do you face in selling your product?
Resources: The first challenge is that the pathology lab needs some investment in digital infrastructure, specifically, they need to have a digital slide scanner that transforms physical tissue biopsies on glass slides into digital images that our algorithm can analyze.
Confidence: Pathologists, like most physicians, would like to see clinical data that supports the accuracy levels of our solution, in other words, how accurate it is in detecting cancer. They need to be convinced that it’s “clinical grade”.
AI is very much hyped nowadays and may be perceived by some pathologists as a threat as if it’s here to replace them. The reality is that we’re here to empower them, help them reduce error rates and make their workflow more efficient so that they can focus their expertise on the most complex cancer cases.
The challenge is to convince them that adopting our solution will lead to these benefits.
What are your predictions for AI in Healthcare and Cancer diagnosis, and even cancer treatment?
AI will first help labs and physicians improve their workflows (eg, triage), optimize their work (eg, CAD- computer-aided diagnosis), and prevent errors (eg, QC). Ibex’s SR system is already deployed in several labs, where it operates as a fully automated QC, or safety net, drastically reducing the rate of misdiagnoses.
In the next couple of years, we expect AI systems to be more widely adopted and to have a more active role in the diagnostic processes. Pathologists and radiologists will not just work digitally and use algorithms to measure and quantify features in the images they examine, but actually collaborate with AI to reach a more accurate, objective and reproducible diagnosis, and do this more efficiently.
The next step will involve two changes:
First, some diagnostic tasks will be fully-automated – AI will perform the entire diagnosis by itself, and the human physician will supervise the process and provide assistance in special cases. The roles of humans and AI will be inverted.
Second, AI will go beyond mimicking the physician’s diagnosis. AI models will integrate data from diverse sources, acting as a sort of tumor board, where experts from multiple fields share their findings and knowledge to decide on the final diagnosis and treatment – AI will be an expert in all these fields, and will have the ability to combine the information more accurately and effectively.
AI will also find new signals in the data, e.g., new patterns in pathology slides or radiology images, that humans have failed to detect so far (eg, since the signals are too small for the human eye, or too complex for the human brain) – these patterns could improve the accuracy and reliability of diagnosis.
Furthermore, AI will be used by oncologists for precision medicine, ie, provide personalized treatment for each patient based on a multitude of data types. For example, AI will combine pathology, radiology, genomic, clinical and demographic data, and using huge databases of medical records, treatments, and outcome, it will stratify patients into smaller, more homogeneous groups, provide an accurate prognosis for each one, and match the best treatment per patient.
What differentiates your solution from others on the market?
Field-proven: Ibex is the only company to have an AI cancer detection solution used in routine clinical practice in pathology and a solution that is successful in detecting misdiagnosed cancers.
Scope: The only company with a solution for the detection of prostate and breast cancers
Accuracy: Ibex’s Galen Prostate solution demonstrated the highest accuracy measured in a clinical study in the field, and was the only solution that’s been compared to independent pathologists in a blinded study, making it a real clinical-grade solution.
How can you ensure your AI is efficient?
Once we are done training an algorithm, for example, one that detects cancer in prostate biopsies, we test it on a new dataset of samples – prostate biopsies, but ones that were not part of the training process. We let the algorithm do what it’s trained to do – detect cancer. Then, we compare the results with the results of the original pathologist that reviewed the biopsy. Whenever we note a discrepancy, we involve another pathologist to resolve who’s right. By the end of this meticulous process we get a score for the algorithm’s performance, its accuracy level if you like. We release an algorithm only when its accuracy levels are very high, typically matching the best human pathologists.
Another way for us to test our algorithms is to run retrospective studies where we let them run on biopsies pooled from independent labs and medical centers. It’s similar to the previous process but done as a full-blown clinical study, with independent pathologists, and with fully blinded data. We have already completed a few such studies in Europe, the US, and Israel, and our solution demonstrated outstanding performance, at least as good as the best pathologists.
Has your solution proven to be successful? Do you have any clients yet?
We already have satisfied customers that use the system, which is the best proof.
Moreover, the solution was already successful in alerting pathologists on misdiagnosed cancers – in other words, patients who had cancer that the pathologist missed. Let that sink in a bit, what it means for a patient to walk around not knowing, and not being able to treat cancer on time, which could sometime be the difference between life and death.
Read More: AiThority Interview with Venkat Rangan, Co-founder, and CTO at Clari
What can we expect from AI in the coming years?
Similar to what I mentioned above on Q5 – AI will become:
– More powerful in several axes – more accurate, surpassing human performance in many tasks
– More general, performing multiple tasks and integrating multiple types of inputs and data sources (text, images, …), rather than focusing on a single, narrow computation on one type of data
– More interconnected, exchanging information and conclusions/findings among AI systems with no human intervention, and more ubiquitous, helping people (including, in our case, physicians), replacing them in some tasks, integrating into many devices and software programs, etc.
What are your predictions for the Medtech industry over the next five years?
Increased adoption of digital technologies with AI becoming a leading driver in the following fields:
– Screening and decision support tools
– Efficient workflows
– Predictive diagnostics and personalized medicine
– Quality control
– Remote working
What’s next for Ibex?
Ibex will introduce new solutions for integrated diagnostics that will help pathologists through the entire diagnostic pathway, improving their accuracy and making their workflow more efficient – helping them improve turnaround times and helping the healthcare system provide better care and reduce costs.
Expanding the scope of tissue we are covering. Currently, it’s breast and prostate – we will add new tissue types in 2020, and even more in the coming years. Predictive diagnostics and personalized medicine solutions that we will develop together with pharma companies.
Thank you, Joseph! That was fun and hope to see you back on AiThority soon.
Joseph Mossel is the CEO and Co-Founder of Ibex Medical Analytics. Over his career he served as a software engineer, product manager and in leadership roles in startups, corporates and non-profits, and has taken products from inception to multi-million dollar businesses.
He holds an MSc in computer science from Tel Aviv University, and an MSc in environmental science from VU Amsterdam.
Ibex uses AI to develop clinical-grade solutions that help pathologists detect and grade cancer in biopsies. The Galen Prostate and Galen Breast are the first-ever AI-powered cancer diagnostics solutions in routine clinical use in pathology and deployed in labs worldwide, empowering pathologists to improve diagnostic accuracy, integrate comprehensive quality control and enable a more efficient workflow. Ibex’s solutions are built on deep learning algorithms trained by a team of pathologists, data scientists and software engineers.
Comments are closed, but trackbacks and pingbacks are open.