AiThority Interview with Dr. Anand Rao, Global AI Lead | AI-Emtech Lead in Labs | Partner in Analytics Insights at PwC
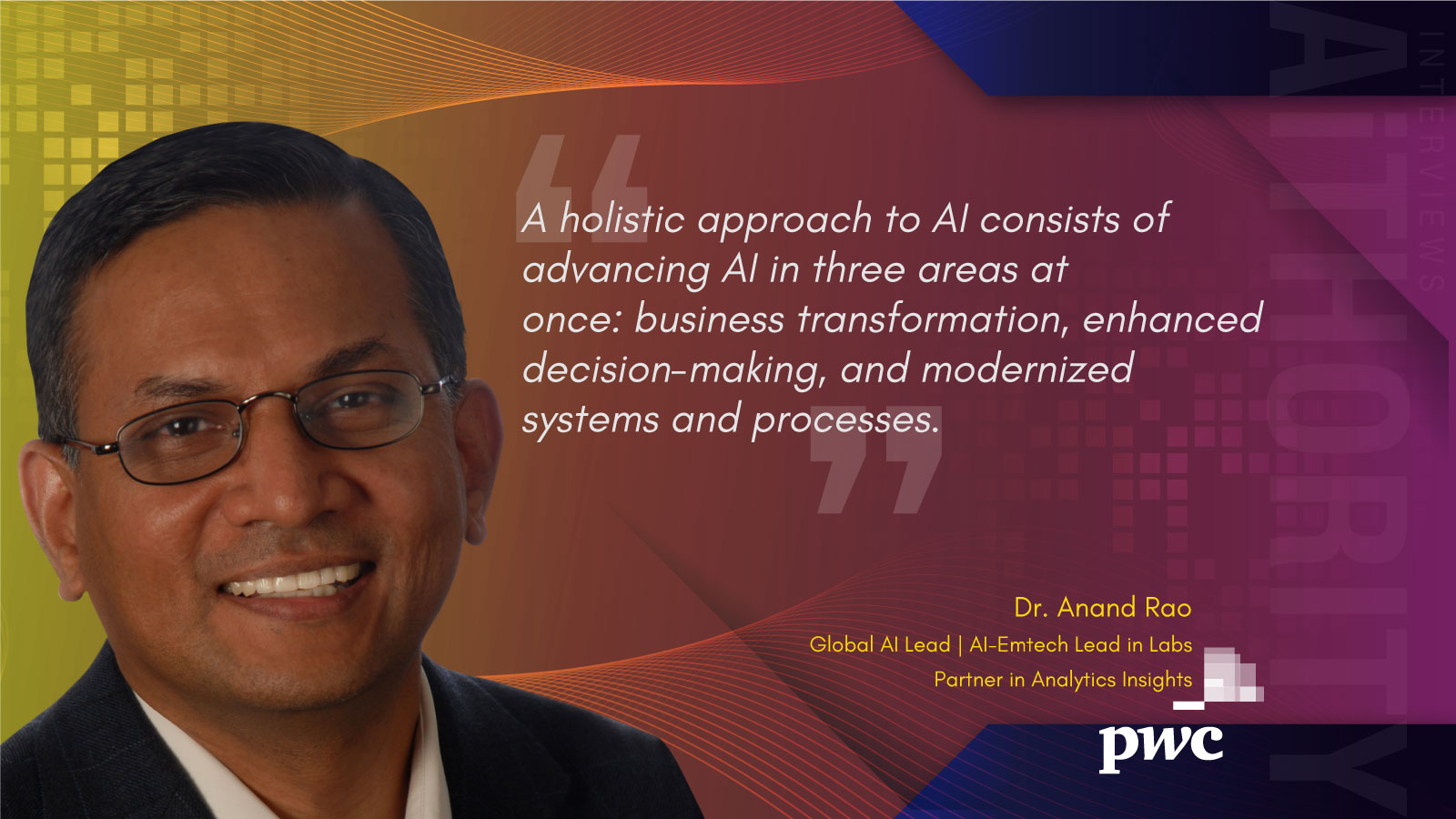
Hi Anand, please tell us about your experience with AI solutions. What is your current role / responsibility at PWC? What kind of AI engineering and product development roadmaps do you focus on?
I have around 34+ years of experience in researching, designing, building, testing, and governing AI solutions. I have spent roughly a third of my career in AI R&D, a third in business strategy, and a third in applying AI solutions to a variety of businesses in banking, insurance, healthcare, technology, media, telecommunications, automotive, aerospace, defense and agricultural sectors.
I currently have three key responsibilities within PwC. I am the Global Artificial Intelligence lead for PwC facilitating and coordinating our global AI initiatives including engagement with government and policy makers on their National AI strategies and enterprises adopting AI solutions. I am also the AI and Emerging Technology leader within PwC’s Products & Technology managing the research, innovation and application of AI, blockchain, AR/VR, Web 3.0 and Metaverse technologies. I am also a Partner in the Analytics Insights practice within our Cloud and Digital platform focused on delivering AI solutions to our clients.
My primary focus in these key roles is to grow the AI capabilities of PwC and our clients in innovative solutions as well as scaling these solutions at the enterprise and national levels. I am responsible for the research and innovation agenda as it relates to AI and other emerging technologies and interact with a number of universities, start-ups, and incubators. I also focus on the roadmap of key products, solutions, and accelerators that have a substantial AI component or solution embedded into it.
Recommended: AiThority Interview with Pete Wurman, Director at Sony AI America
PwC recently released a powerful AI Machine Learning report for business leaders. Could you please explain for the benefit of our readers, what you mean by “taking a holistic approach to AI”?
When developing an AI strategy, AI leaders tend to focus first on one goal, then move on to the next. A holistic approach to AI consists of advancing AI in three areas at once: business transformation, enhanced decision-making, and modernized systems and processes. Our recent 2022 AI Business Survey found that the companies that are taking this more holistic approach to AI and prioritizing these three goals tend to see greater success than businesses taking a singular approach. In fact, according to the survey, a third of leaders reported widespread AI adoption spurred from taking a holistic route.
This AI approach brings together leaders from across the organization and facilitates scaling and data sharing. It gathers AI specialists, analytics teams, software engineers and data scientists to help better align on goals for AI projects. Not to mention, it can also help set businesses on the right track for their projects to deliver a real impact at a reasonable cost.
Perhaps the most critical part of taking a holistic approach is that it requires the investment in and management of data, AI and cloud as a unified whole. AI delivers the most value at scale, embedded in application systems that are analyzing data nonstop. These systems can only be effective, however, when cloud computing is leveraged to scale up and down. Leaders who prioritize a holistic approach to AI can take advantage of this.
How do you see newer technologies such as AI Optimization and AutoML further improving product offerings in the coming months?
AI Optimization and AutoML product offerings are democratizing the use of AI and data science. Optimization is a highly mathematical and statistical discipline requiring deep expertise. AI Optimization offerings seek to standardize the algorithms by specific optimization domains to facilitate greater adoption e.g., pricing, logistics, resource optimization. A large number of machine learning techniques rely on better optimization techniques to improve their performance. Deep learning architectures, especially large foundational models with billions and trillions of parameters, require advanced AI optimization to be viable solutions.
Similarly, AutoML offerings partially automate the AI and data science model design, development, and testing of AI/ML models. AutoML offerings not only increase adoption of AI to a larger group of power users and data scientists, they also add consistency, auditability, and automation to the model management lifecycle. AutoML solutions also facilitate better governance of AI/ML models and are often the first step towards Responsible AI. However, excessive democratization of AI without the necessary training and education could be counter productive. Businesses should balance their adoption of AI Optimization and AutoML offerings with extensive training of their data scientists and instituting appropriate governance for data and AI/ML models.
Automation seems to be the number one use-case for most AI customers. Why do you see companies still lagging in their ability to successfully implement AI and data science for acquiring superior agility and resilience in their business operations?
While AI provides businesses with a host of benefits, the truth is that the road to successful ROI takes time. There are numerous barriers in adopting the technology. More often than not, the biggest challenge is recruiting and retaining AI talent: 79% percent of companies are slowing down AI initiatives because of limited AI talent. AI and data science specialists are not only scarce but they’re often expensive, hindering the roll out of AI for businesses.
In addition to the core AI and data science talent companies often lack “bi-linguals” or domain experts who understand AI and data science who can help in scoping and directing the more junior data scientists. This skill is harder to acquire and needs to be grown organically further challenging the successful implementation of AI solutions.
The first step to retain valuable AI talent is to keep current employees happy. Reduce the need for rote work and give workers the opportunity to train with cutting edge AI, increasing both the value you’re providing them and the value they can give you. Adding to this, investing in data-driven people is key. Businesses can address the AI talent shortage by taking specialists who have some necessary skills — then providing the rest. For example, consider teaching computer scientists data science, and vice versa. Not only will this help businesses address AI shortcomings but it will also enhance cooperation among all business units.
I would argue that AI is also heavily being used in other major use cases beyond automation. One major use case is to provide predictive analytics creating powerful forecast models, predictive maintenance and predicting user behaviors. Another major use case is in turning unstructured data, like text, voice images, and video into actionable insights. This is dramatically increasing the volume of data which can enable better decisions and modernizing processes that interact with people and machines. These are large scale uses of AI which are in production across a wide range of industries.
Recommended: AiThority Interview with Alex Springer, VP EMEA – Sales and Solutions Architects at impact.com
Do you think AI helps to improve and sustain investors’ and stakeholders’ trust in the company and its products? What does PwC say about this in the report?
Over the last 12 months, 15% of leaders have prioritized enhancing stakeholder trust via AI initiatives. That is a relatively low number considering our survey also found that 98% of leaders have at least some plans to make their AI responsible in 2022.
When effective, responsible AI governance offers checks and balances, as well as escalation protocols when evaluating AI models. For this reason, stakeholders and investors are likely to have many questions about an organization’s use of AI and data, especially how it is governed. Businesses need to be ready to provide answers, and demonstrate ongoing governance and regulatory compliance.
While nearly every company seems to have responsible AI ambitions, fewer than half have plans to address issues of fairness. Leaders taking a holistic AI approach may be doing better on this governance, but there is room for plenty of improvement in the years to come.
Which industry, according to you, should increasingly focus on inclusion of more data-driven innovations, particularly AI and Cloud?
All industries can benefit from AI and cloud initiatives. Let’s use supply chain as an example, given the current challenges in the sector. Currently, 34% of businesses are using AI to make decisions about their supply chains and 41% of companies are using AI simulations to enhance supply chain and operations.
The supply chain market can benefit from the use of AI simulations, such as digital twins, in particular. These are powerful in that they can provide detailed, real-time insights into current performance. For example, when bringing together simulations of suppliers, customers, and even the weather, operators can more accurately predict pricing dynamics and distributions, getting ahead of the curve to navigate any potential upcoming challenges.
AI is making inroads in other verticals as well. Thirty-two percent of companies are using AI to make workplace decisions that include DE&I and 11% are leveraging it to determine ESG standards for company operations. For example, an estimate of an investment’s carbon footprint can be more precise when AI models are used to project future energy supplies. Ultimately, businesses across industries are beginning to think better, faster and longer term through their decisions powered by AI support.
What would you say about the IT infrastructure that an organization requires to successfully transition into a fully-AI focused entity?
Organizations that want to transition into a fully-AI focused entity need to focus on bringing three related disciplines of data, models, and software together. This requires them to change their IT infrastructure, their design and development process, and the skills and talent of their people. Having access to the right data is essential for AI programs, and data readiness can often make up more than 85% of the effort on AI.
A modern data fabric for the enterprise makes data more accessible for AI. The IT stack of an AI-focused entity typically includes an AI or insights tier between the data layer and application layer. This allows AI/ML models to be used both on a standalone basis as well as being embedded into traditional application software (e.g., GL or finance, customer relationship management, supply chain management).
In addition, AI/ML models would require data pipelines to be built for continuous learning (CL) of models. CL needs to be integrated with continuous integration/delivery (CI/CD) of software. AI-focused infrastructure should allow for development and production environments. The production environment should include Data Ops, MLOps, Security Ops, in addition to the traditional DevOps. The IT infrastructure should be scalable with on-prem and cloud infrastructure for storage and compute.
Your take on the skills gaps in the data analytics and AI markets—what’s your advice to the hiring managers and business leaders when it comes to tapping best AI minds?
Companies face two types of skill gaps – one focused on AI and data science technical talent and the other focused on “bi-lingual” or “multi-lingual” mid-level managers and business talent. The second type of talent are business domain experts who also understand data science.
Multi-lingual managers bring domain expertise, data science expertise and also data and/or software expertise. Hiring a large number of such bi-lingual and multilingual talent from outside is a significant challenge. Having a robust training and mentoring program to enable domain experts to learn data science, software and data engineering is key to growing and retaining this talent. Democratization of data science, data, and AI/ML ease the transition of this training and education.
Recommended: AiThority Interview with Sam Yoshida, EVP & GM of Canon USA
Your predictions for the fully-managed AI-As-As-Service architectures in 2022?
Fully managed AI-as-a-service is still in its infancy. As vertical applications in financial services, healthcare, retail etc. gets more standardized and horizontal applications in contact centers, supply chain, customer service gets standardized we will see more of an opportunity for managed services. We see contact center, customer service, and customer experience use cases that integrate IVR, contact center, human chat, and chatbot/conversational AI as the area that is well positioned for a fully-managed AI-as-a-service. Contract management and social media/external content management are also ripe for a fully managed AI-as-a-Service solution in 2022.
Thank you, Anand! That was fun and we hope to see you back on AiThority.com soon.
[To share your insights with us, please write to sghosh@martechseries.com]
Anand Rao is a Principal with PwC’s US Advisory practice, with over 24 years of industry and consulting experience, helping senior executives structure, solve and manage critical issues facing their organizations. He has worked extensively on business, technology, and analytics issues across a wide range of industry sectors including Financial Services, Healthcare, Telecommunications, Aerospace & Defense, across US, Europe, Asia and Australia. His work has included behavioral economics, simulation modeling, global growth strategies, marketing, sales, and distribution strategies, online, mobile, social media strategies, customer experience, multi-channel integration, risk management and compliance, large scale program mobilization and management. Before his consulting career, Anand was the Chief Research Scientist at the Australian Artificial Intelligence Institute, a boutique research and software house.
At PwC, our purpose is to build trust in society and solve important problems. We’re a network of firms in 155 countries with over 284,000 people who are committed to delivering quality in assurance, advisory and tax services. PwC refers to the PwC network and/or one or more of its member firms, each of which is a separate legal entity.
Content on this page has been prepared for general information only and is not intended to be relied upon as accounting, tax or professional advice. Please reach out to your advisors for specific advice.
Comments are closed.