AiThority Interview with Taejin In, VP of Product Management at dstillery
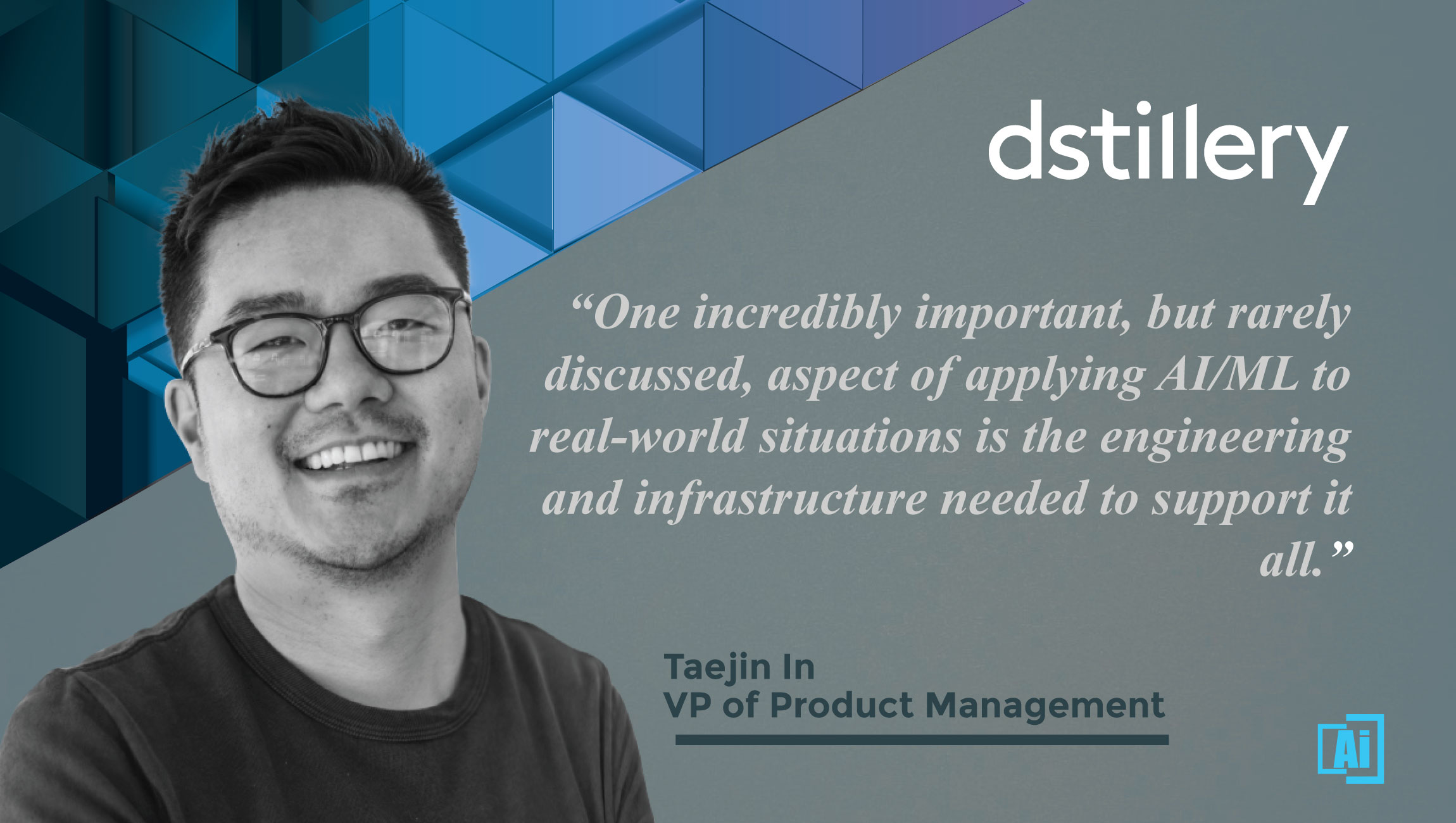
Tell us about your role and the team/technology you handle at Dstillery.
I lead product organization at dstillery. Our team has three technical areas that we oversee from a Product Management perspective: audience studio, audience scoring, and audience data and syndication. We also keep tabs on developments and innovations around new audiences types, data quality, data types, and generally better and more efficient ways to do things. These innovations are often identified outside the product organization, from technical teams (Data Science, Engineering) to business teams (Sales, Business Development), and it’s our job to codify the learnings into items for our roadmap.
Why are B2B Marketing teams steadily moving toward Applied Data Science for Sales and Marketing initiatives?
We see Marketing teams doing this because it works, and it works across two factors. One, applying AI and Machine Learning to Marketing initiatives drives enhanced performance for campaigns, whether it’s used for optimization-type functions or for decision-making/strategic planning functions.
The other factor is the removal of repetitive and error-prone processes from one’s day-to-day work process. This lets marketers focus on areas where they can add more value — things like creative endeavors, calculated risk-taking, and relationship/partnership building, just to name a few.
Tell us about your product, Audience Studio. How can MarTech customers benefit from your platform?
Audience Studio is a self-service, end-to-end platform that allows users to explore, create, activate, and map their audiences. Working with marketers for over a decade, we’ve identified the key areas where marketers extract the most value from our proprietary data, Machine Learning, custom audience building, and activation capabilities.
We’ve built an easy-to-use interface so that our customers can do what we’ve done for them in a self-service matter. We’ve taken some Marketing functions that normally require intensive work from a team of humans (like building custom AI models, or generating insights reports), and condensed them down to a few clicks.
Read More: AiThority Interview with Massimiliano Versace, CEO and Co-Founder at Neurala
What is the current state of Audience Data Analytics?
The analytics space is improving but could be much better. The walled gardens are getting good at providing transparency and audience data analytics as added value to customers, which I think is a function of it being good business for them. I haven’t seen too many great audience data analytics solutions for the open web.
The various platforms, DSP and DMPs all have some sort of version, but of course, it’s not their core business, and you can tell. Also, audience data analytics is only as good as the data that powers it. I’ve seen some instances where the data is a bit hazy, which makes the analytics that come out even hazier.
How much of this state is influenced by the maturity of Data Science, AI, Automation and Content Personalization?
Not as much as is possible. The application of Data Science, AI, and Machine Learning, in terms of audience data analytics, is still in its infancy. A lot of the analytics I see are based on simple overlap calculations, or the most basic logistic regression modeling at best. This makes me hopeful, though, as there’s so much room for growth and improvements across the category.
I see some issues with turnaround times as well. As companies get better at fusing Machine Learning with their core engineering disciplines, they will get better at providing analytics quickly, as timeliness is of the utmost importance in the Marketing industry.
What are the major pain points for Product Management and Innovation teams in meeting the business demands?
A lot of the pain points come down to directly measuring the impact of AI-based technologies on revenue. Technology is just one lever among dozens (maybe hundreds) of things that impact the success of a campaign. Without successfully assessing the causal impact of these new features and innovations, it’s hard to specifically pinpoint what worked and what didn’t. This data is incredibly important to ensure you’re making the right investments in the right places.
How can we solve the challenges in delivering personalized feeds to customers across platforms?
In terms of content personalization, I think there’s a huge opportunity to leverage the technology stack that’s been built for programmatic advertising and applying it to a non-direct advertising use case. For example, pretend that a piece of content is the same thing as a piece of digital advertisement.
You can apply all the technology that enables programmatic ad buyers to show the right ad to the right person at the right time, and instead, use it to show the right piece of personalized content to the right person at the right time.
Read More: AiThority Interview with Richard Jonker, VP of Product Management (SMB Products) at NETGEAR
When can we see better adoption of Video Marketing tools for Email Marketing and Mobile Messaging? Or, is it just too much to ask for in 2019?
AI-driven growth seems to happen in areas that seek the two things I mentioned earlier: A desire to drive much better performance, and a desire to free humans from doing the optimizations so that they can focus on other things. When those two desires become absolutely paramount, then the AI-based solutions will follow.
How do you work with AI/Machine Learning at dstillery?
AI and Machine Learning are the building blocks behind Audience Studio and dstillery’s audience-building technology. We use sophisticated AI/ML techniques that learn the nuances of audience behavior, and what consumption these behaviors drive. Also, we’ve found success with a variety of optimization algorithms, including stochastic gradient descent, because our space relies on fast tuning to deliver successful campaigns. One incredibly important, but rarely discussed, aspect of applying AI/ML to real-world situations is the engineering and infrastructure needed to support it all.
Dstillery has built a system that maintains as many behavioral attributes as possible for every device. This could mean millions of features for hundreds of millions of devices. On top of that, we re-score users into audiences every single day. This is a mind-boggling infrastructure and algorithmic challenge, but it’s worth the investment as it maximizes the predictive quality and accuracy of our audiences.
Thank you, Taejin! That was fun and hope to see you back on AiThority soon.
Taejin In is Vice President of Product Management at dstillery. He was previously the Co-Founder of Krave Group LLC.
Comments are closed, but trackbacks and pingbacks are open.