AiThority Interview with Bret Greenstein, Partner, Cloud & Digital – Analytics Insights at PwC
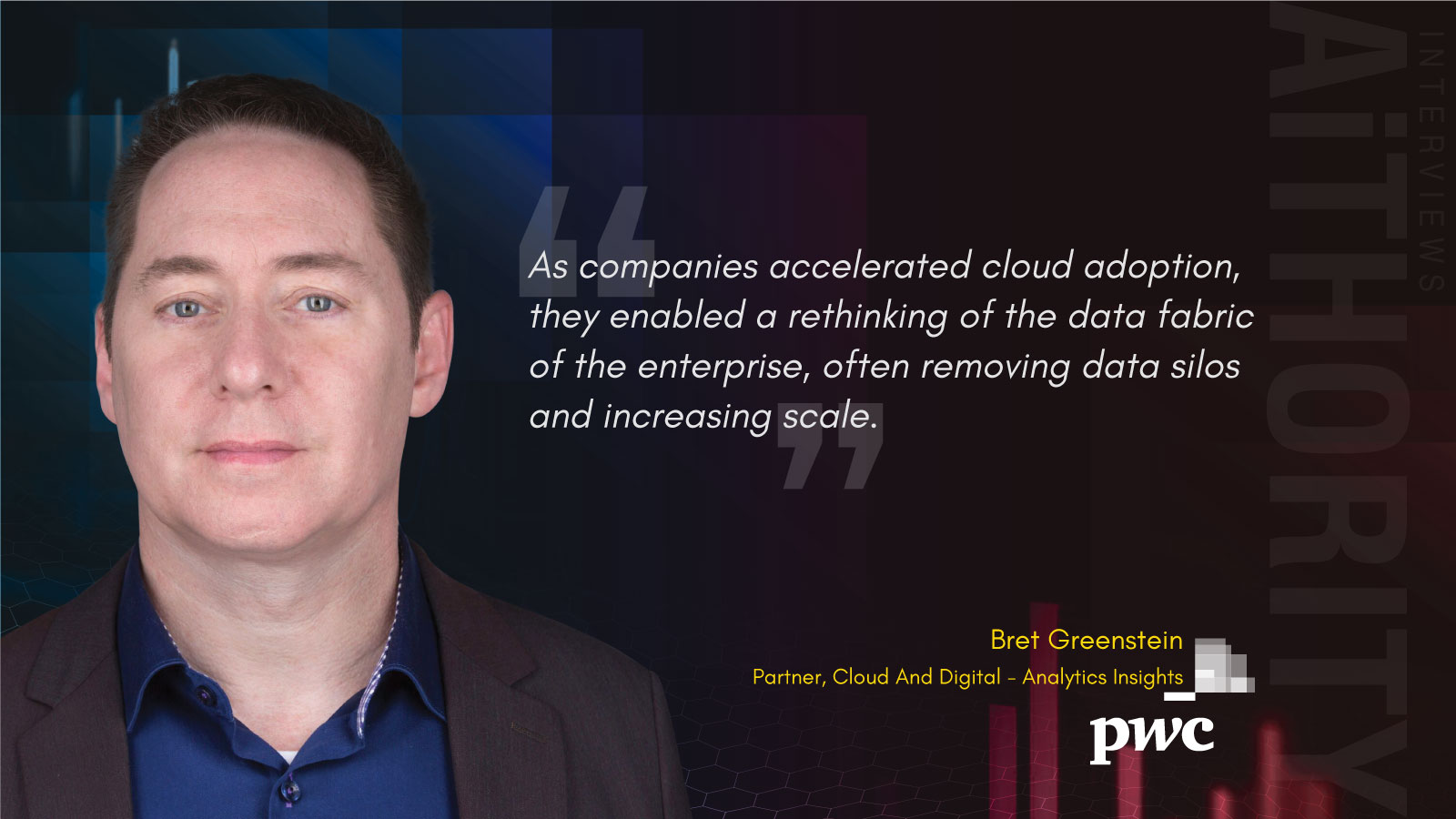
Hi Bret, please tell us about your experience with data analytics and AI solutions. How have data analytics trends evolved since the time you first started in this industry?
I have been involved in data and analytics for my entire career. Starting out in semiconductor manufacturing, I was amazed by and inspired by the power of data in understanding manufacturing efficiency, product yields, equipment performance, and a wide range of operational decisions in running a manufacturing line. Semiconductor manufacturing has been complex for a long time and was an early user of “big data” given the complexity of the products being made and the complex machines, chemicals and environment used in manufacturing.
It shaped my views on a wide range of data topics. I was using forecasting models and simulations, and running sensitivity analysis on changes in processes to predict how parts would come out. We supported daily and hourly operations processes that decided on what to run, and how to optimize the flow of parts through the factory.
These all feel like the patterns we see today in supply chains, healthcare, and banking with data and analytics. While those early days involved tedious, complex queries and spreadsheets on large on-prem servers, the patterns have continued to be relevant today. As the underlying technologies became easier to use and scale, the ways data is used haven’t changed that much. It is just available to a lot more people and more use cases than ever before.
What is your current role / responsibility at PwC? What kind of AI engineering and product development roadmaps do you focus on?
I lead large scale transformations for clients using data, analytics and AI on cloud. This involves everything from helping clients to establish strategies for their business based on data, analytics, and AI, to developing roadmaps, building out architectures, and running build/integration/test of new environments for clients. I also work in partnership with other teams driving business transformation which is all heavily dependent on data, analytics, and AI now. I am especially focused on helping clients to use aata and AI more effectively, how to govern them, ensure quality, recognize and address bias, and ensure data and AI deliver business outcomes.
Recommended: AiThority Interview with Amy White, Director of Social Impact and Communications at Adobe
PwC recently released a powerful AI report for business leaders. Could you please shed some light on the findings of your research and how businesses are increasingly expanding their budget for AI adoption?
PwC’s fourth annual AI Business Survey shows AI is a tool businesses can’t do without. The companies with the most ROI are those that don’t focus on one goal at a time, our research found. Instead they are advancing AI in three areas simultaneously: business transformation, enhanced decision-making, and modernized systems and processes.
A few more specific findings from the survey include:
Companies that have implemented a three pronged approach to achieving their company goals through AI are using it to make decisions about technology (50%), about operations and maintenance (34%), and about customer experience (40%). Soon I anticipate AI will be used to solve more complex business decisions, like with ESG.
The survey also found responsible AI is a priority for 98% of businesses, but they disagree on the best way to implement this. For example, 40% plan to protect AI systems from cyber threats and manipulations, while 40% plan to confirm AI is compliant with applicable regulations. Either way, it’s an area of focus to improve here.
What are AI leaders doing right? Which areas cause the maximum trouble for lagging parties in adoption of AI capabilities?
AI leaders are taking a collaborative approach to AI and are bringing together their top performers from across the organization. These include AI specialists on analytics teams, software engineers, and data scientists. This unified approach facilitates scale and data sharing and helps align outcomes with business priorities, leading to organizational buy-in and to projects that deliver real impact at a reasonable cost.
To further predict and boost AI’s ROI, leaders are using a data-AI-cloud (DAC) life cycle rather than managing individual AI projects. This helps them to evolve strategies, fine-tune execution and find new use cases for data.
In order to keep up with the rapid advancements in AI, it’s important for leaders to deploy end-to-end governance of the DAC life cycle. Risk, artificial intelligence, and business leaders all need to be integrated with the new procedures, roles, and responsibilities for each line of defense.
Meanwhile, recruiting and retaining talent is causing the most trouble for companies looking to adopt AI as AI talent is often scarce and expensive — 79% of companies are either slowing down AI initiatives or developing a strategy to do so due to a lack of AI talent. Yet companies taking a holistic approach to AI are far more likely to be continuing full speed ahead with AI initiatives despite the AI talent shortage.
Compared to companies that approach AI in a piecemeal manner, companies taking the comprehensive approach are more often focusing on retraining in-house talent to work with AI (43% versus 33%), and reorganizing teams to make better use of limited AI experience (41% versus 32%) to close this skill gap. In addition, businesses are increasingly turning to third parties, such as managed services providers, with their scalable and multifaceted AI workforce, to support AI initiatives.
Could you please explain the difference between the “piecemeal approach to AI” versus wider adoption approach? What is PwC doing in this area to enable faster and agile business transformation for AI prospects?
For a long time, AI projects, and data science in general, were done in islands across the enterprise. This often led to poor results either because the projects were not sponsored by business leaders who understood AI, or because these teams didn’t have the full access to the data that was required.
What I advocate for with clients is to ensure business sponsors are in place and are enabled on the potential of AI and data, and that they team up with IT teams and Chief Data Officers to ensure data access and quality in support of AI. It is only when these really come together that companies get scale, benefits, and enable growing innovation with data. Data is meant to be unlocked and leveraged by AI for business outcomes.
Recommended: AiThority Interview with Nithinan Jessie Boonyawattanapisut, Co-CEO & Director at NextPlay Technologies
How have AI and big data management roadmaps evolved in the last 2-3 years, particularly with the rise and popularity of Open Source devops communities?
The biggest changes in the last few years have been:
- Cloud, cloud, cloud. As companies accelerated cloud adoption, they enabled a rethinking of the data fabric of the enterprise, often removing data silos and increasing scale.
- Agile sprints towards a strategic future. More companies are creating enterprise data and AI strategies to ensure there is a clear vision with business buy in. Under that strategy, they are encouraging innovation with data and are driving incremental movement towards the strategy architecturally. I see companies filling in the strategy in sprints, delivering value along the way, building buy-in, and educating and enabling wider adoption of data and AI in the enterprise.
A business domain that you think is benefitting / would benefit the most from AI’s transformative role?
Right now, at least 50% of businesses are using AI to make any number of decisions, for everything from operations to product development. AI-supported decision making is powerful because it enables leaders to incorporate and analyze more information than any human being could do on their own.
I anticipate we’ll start to see more companies use AI to make complex business decisions around ESG, the workforce, and more. Right now, only 9% and 24% of organizations respectively use AI to support these decisions, but there are some great complex use cases available to businesses. In ESG, for example, a single facility’s carbon footprint can be calculated more accurately when AI models are used to forecast weather patterns or future energy supplies.
AI analysis also has a lot to offer in M&A as well, which currently only 16% of businesses are using AI for. By leveraging AI, companies can automate certain aspects of due diligence, predict regulatory responses and project combined company results under various circumstances.
An AI-centric industry you are keenly following now, and why?
I am fascinated by everything that AI touches today. From cars to toys to decision system. I look for signs of AI being used to forecast, design products, engage with people, assist people in customer service, drive cars, and creating images, videos and text. I always imagined AI would have wide reaching impacts, but I never thought I would see so many things touched by AI so quickly over the last 5 years.
Which industry you think should increasingly focus on inclusion of more data-driven innovations, particularly AI and Cloud?
The adoption of cloud and AI is now universal across industries. I think it will be compelling to see AI really transform customer service so that every interaction you have with a brand is the smartest and most personalized and informed interaction possible. I also want to see consumer products continue to adopt AI to demonstrate better understanding of our wants and needs, of the environment around them, and of the best ways to solve problems.
What would you say about the IT infrastructure that an organization requires to successfully transition into a fully-AI focused entity?
It is critical that enterprises increase the democratization of data and insights, improve discoverability, embrace external data, and make it easier for people to get access to the data that matters most to their roles. Too many companies still operate with data silos and islands, and are not encouraging expanded use of AI across the business.
Your take on the skills gaps in the data analytics and AI markets—what’s your advice to the hiring managers and business leaders when it comes to tapping best AI minds?
There are a lot of people pursuing the right skills. But there is never enough of them. Clients, service providers, and technology platforms all need more people. The good news is that I am seeing people pick up data science and engineering even late in their careers. The technologies are new enough that everyone needs to continue to learn.
Recommended: AiThority Interview with Scott Brinker, VP Platform Ecosystem at HubSpot
Your predictions for the fully-managed AI-As-As-Service architectures in 2022?
AI-as-a-service is not really where I see the market in the near term. I do see specialized AI services being available, for example machine vision or text generation, but these are narrow and specialized and are really more like super-APIs than they are truly AI-as-a-service.
How do you see newer technologies such as AI Optimization and AutoML further improving product offerings in the coming months?
These are very exciting areas. They make the work of data science more automated and accessible to a wider range of technologies. Using AI to help create and grow AI is absolutely the future. Making AI easier to create, manage, and improve will make AI a more inclusive career path to a wider range of people who aspire to work in the field.
Thank you, Bret! That was fun and we hope to see you back on AiThority.com soon.
[To share your insights with us, please write to sghosh@martechseries.com]
Bret leads PwC’s US Data and Analytics Strategy and Alliances. He has worked for over 25 years helping clients to transform through the adoption of new technologies, including AI, Data, Internet of Things, and Cloud, to deliver new business models and new ways of working. He joined PwC from Cognizant where he was the SVP and Global Head of AI and Analytics. Prior to that, he worked in IBM as a P&L leader for multiple software and services brands, and he was the CIO for IBM’s Growth Markets based in China. Bret brings a focus on scaled transformation using AI, Analytics and Data with cross-industry experience working with clients all over the world. His business and technical
experiences have helped him become a trusted partner for clients trying to accelerate their digital journey. Most recently, Bret has led the data strategy and transformation programs at major industrial clients. In this work, he helped them to develop data driven business strategies, creating a data fabric for the enterprise which supported finance, supply chain, commercial, HR and manufacturing transformations. He emphasizes business driven data governance and architecture, and on helping clients to understand how to use AI to transform decision making.
At PwC, our purpose is to build trust in society and solve important problems. We’re a network of firms in 155 countries with over 284,000 people who are committed to delivering quality in assurance, advisory and tax services. PwC refers to the PwC network and/or one or more of its member firms, each of which is a separate legal entity.
Content on this page has been prepared for general information only and is not intended to be relied upon as accounting, tax or professional advice. Please reach out to your advisors for specific advice.
Comments are closed.